An Investigation into the Feasibility of Accurate Lake Surface Temperatures
Research Topic:
Data Fusion and Algorithm Development
Task Leader:
Andy Harris
CICS Scientist:
Andy Harris
Sponsor:
STAR Ocean Remote Sensing
Published Date:
9/5/2017
2017 ANNUAL REPORT
Background
NESDIS has long provided surface temperatures for the Great Lakes region as part of the CoastWatch suite of products. However, there has been no specific attempt to tailor these products to ensure that accuracy and coverage requirements are being met. Furthermore, there are many other lakes in North America for which end users desire accurate surface temperatures, but which have received scant attention. One prime indication of the need to increase work in this area is the User Request put in by NWS to the NESDIS Satellite Products and Services Review Board some two years ago that stated their need for accurate lake water temperatures. In their User Request, NWS went on to outline some of the problems that they perceive to exist in current lake surface temperature products, including inadequate cloud masking, biases due to anomalous atmospheric conditions, and water turbidity. Similar issues are highlighted in reports of the GHRSST Inland Water Working Group (e.g. GHRSST, 2012). Most of the problems in existing NESDIS satellite-based lake temperature products are due to the simple fact that the processing methodologies for cloud detection and temperature retrieval (e.g. Advanced Clear-Sky Processor for Oceans, ACSPO) have been optimized for the open ocean, where the atmosphere is usually close to equilibrium with the water surface, and the target is far from the disrupting influence of land.
The project evaluates the feasibility of utilizing the ACSPO product for lake surface temperatures. This initially requires the evaluation of ACSPO level-2 data over selected lake targets in North America. A subset of these are lakes for which suitable validation data exist (mostly in situ buoy measurements, such as are present in the Great Lakes). The key metrics that need to be evaluated are coverage and accuracy. Since the ACSPO cloud mask has not been optimized to function over inland water targets, the feasibility of relaxing the cloud mask in order to obtain adequate coverage is investigated. Such approaches usually result in a trade-off in accuracy, which can be evaluated by validation against in situ, where such data exist. Reduction of the cloud mask is likely to require at least some additional filters to be utilized in order to avoid excessive contamination. Furthermore, suitability of the ocean-trained temperature retrieval algorithm under genuinely cloud-free conditions can be assessed. Finally, the prospects for optimizing temperature retrievals for lake water temperatures should be assessed.
Accomplishments
An obvious first step is to assess how much tuning is likely to be necessary in order to adapt the current ACSPO Level 2 temperature product to provide usable lake temperatures (i.e. adequate accuracy and coverage). Following on from this, initial investigations into the prospects for improving both of these aspects should also be investigated.
Methodology
The primary purpose of this project is to assess the feasibility of adapting existing data products (ACSPO) to obtain lake water temperatures that meet coverage and accuracy requirements. In order to accomplish this, a number of tasks eventually need to be performed:
1) Establish a high-resolution land/lake mask that identifies an appropriate range of lake targets in North America. These targets should include lakes of appropriate size range and representative distribution across differing retrieval environments (altitude, continental & maritime environments, etc.). Some lakes need to contain validation data in order to assess product accuracy. All lakes can be used to assess coverage performance (i.e. frequency of obtaining temperatures that are deemed cloud-free);
2) Perform matches of ACSPO swath data against the lake targets and analyze statistics, including samples of accuracy of geolocation, percentage of lake that is deemed cloud-free, and range of temperatures within each lake (an indication of structure, which is a measure of how important the % coverage metric – lakes which are uniform in temperature need only be partially clear in order to obtain a representative temperature);
3) Assess increase in coverage when relaxing the ACSPO cloud mask, and the concomitant impact on accuracy of the lake temperature retrievals; and
4) Investigate possible ways to improve the product, such as application of tailored QC filters to mitigate the effect of increased retrieval error with a relaxed cloud mask. Such effects are likely to be regional and seasonal. Also investigate prospects for reducing error due to anomalous atmospheres (e.g. cool lake surrounded by warm land, or vice versa), emissivity differences, diurnal warming (water turbidity, wind speed, insolation). In this regard, addition of NCEP NAM surface and upper air data to the matches that include in situ validation will be invaluable. This will allow testing of the MTLS retrieval algorithm to see if local atmospheric conditions can be accounted for. The MTLS algorithm is also quite tolerant of other errors, such as residual cloud.
Sample Findings
The most important aspect of investigating suitability of the current ACSPO VIIRS product for lake surface temperatures is to assess the coverage. If good data which can provide valid observations are being erroneously masked by a cloud detection scheme that has been designed to function over the open ocean, the question of algorithm retrieval accuracy becomes moot. In such circumstances, it might be possible to relax the data quality in order to allow more good observations through, although care must be taken to ensure that bad data are still excluded.
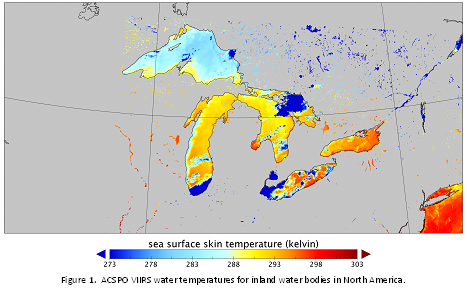
Figure 2 shows an example of ACSPO VIIRS temperature retrievals for the Great Lakes and nearby inland water bodies. It is straightforward to discern by eye that, while there are some cloud-contaminated observations, much of Superior, Michigan, Huron and Ontario are cloud-free and, even with a 30 kelvin stretch, characteristic geophysical fluid dynamics patterns are visible in all of these lakes. The corresponding quality masking is shown in Figure 3, with “good” data shown in white, “bad” data shown as dark red, and some “suspect” pixels shown in a lighter red. The most obvious feature is the masking of virtually all cooler water as “bad”, such as the majority of Lake Superior, which has a characteristic thermal bar with only the southern shore waters getting significantly above ~280 K. The cooler water on the western side of Lake Michigan is also thereabouts entirely masked as “bad”. Furthermore, it is evident that relaxing the quality threshold to allow “suspect” data through has little impact on cov erage, while relaxing further to allow “bad” data also permits significantly cloud contaminated observations (e.g. the cloud bank at the southern end of Michigan). Finally, it can be seen that the vast majority of the smaller lakes to the north of the Great Lakes are completely masked as “bad”.
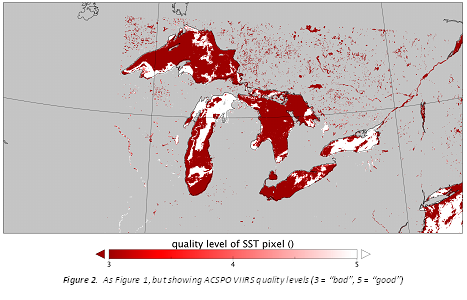
In addition, preliminary work has been performed on investigating the coverage statistics for a modest-sized lake target. This has involved collection of ACSPO v2.4 L2P products (i.e. the current algorithm) from May 2015 – end-2016. The results for retrieved temperature and standard deviation can be seen in Figure 3. The comparison for temperatures using QL=4 and QL=5 are shown. It can be seen that, not unexpectedly, the QL=4 temperatures display significantly more variability, but do add somewhat to the coverage. The retrieved temperatures from the two quality levels do, however, track each other. While the overall seasonal pattern is certainly geophysical, the shorter-term variability (and increased noise) may not be. It is also notable that there is a period around winter (approximately 3 months’ duration) where there are almost no observations. While it might be expected that this would occur when the lake is frozen, the observation frequency does not pick up until the retrieved temperature is at least ~5°C.
Summary
It is clear that using only data defined with a quality level of “good” results in the exclusion of large areas of observations that are actually valid. This exclusion is both substantial and asymmetric, i.e. use of “good” data results in coverage that is both poor and biased warm with respect to the full distribution of valid temperatures. In order to allow the many good observations of cooler water, it is necessary to relax the quality level to include data flagged as “bad”. Unfortunately, this means that truly cloud-contaminated observations are also allowed to pass the threshold.
Although the ACSPO VIIRS products have been developed for the open ocean, it might have been hoped that they would still function fairly well over large bodies of water such as the Great Lakes. It is quite evident that this is not actually the case, and there is not a simple solution. With such poor performance over large water bodies, the lack of coverage observed over smaller lakes was perhaps inevitable. Optimization of the cloud detection will be the first step in obtaining a viable automated lake surface temperature product from the VIIRS mission.
Planned Work
Below are the planned activities on this project, subject to ongoing funding. Additional progress is anticipated on some of the following tasks, since they are being undertaken/completed this year (i.e. intended by end-June 2016)
-
Accuracy metrics for sample targets
-
Feasibility of adapting existing ACSPO cloud masking
-
Investigation of feasibility for localized adaptation of processing
Products
-
Report on feasibility of lake surface temperature product from Polar Orbiting sensors.