CISESS Seed Grant: Machine Learning-based Hyperspectral Sensor Data Retrieval at the CISESS Remote Sensing Laboratory
April 23, 2025 01:59 PM
Figure 1: Artists rendition of PACE satellite
© (Image credit: NASA) from: https://www.eoportal.org/satellite-missions/pace-mission.
Xi Shao, Research Scientist, UMD/CISESS/ESSIC
The advancements in Earth observation and environmental science are driving the need for remote sensing instruments with higher spatial, spectral, and temporal resolutions. . Hyperspectral imaging (HIS) is a process that divides light into many narrow, contiguous spectral bands across the electromagnetic (EM) spectrum, typically between visible and infrared wavelengths. Different features on Earth’s surface reflect, absorb, scatter and emit light at specific wavelengths, which creates a spectral “fingerprint,” which hyperspectral imagers are able to identify. HSI has become essential for identifying atmospheric constituents, and monitoring greenhouse gases, land surface changes, air quality and marine ecosystem. Both Low Earth Orbit (LEO) and Geostationary (GEO) satellites increasingly utilize hyperspectral sensors. NASA’s Plankton, Aerosol, Cloud, ocean Ecosystem (PACE) Ocean Color Instrument (OCI)was launched in January 2024 and delivers hyperspectral data with over 200 bands, providing insights into seawater composition.
This CISESS Seed Grant Project will expand the work of the student-oriented CISESS Remote Sensing Laboratory by performing field experiments with lab hyperspectral radiometerand developing Machine Learning (ML)-based satellite hyperspectral sensor data retrieval capability. The project will be led by ESSIC/CISESS Scientist Xi Shao, along with Wenhui Wang and Tung-Chang Liu. The seed grant project aims to leverage PACE OCI hyperspectral data to develop ML-based retrieval algorithms tailored for hyperspectral missions. Both supervised and unsupervised ML models will be developed to analyze PACE OCI hyperspectral data for ocean composition classification and algae monitoring. Convolutional Neural Networks (CNNs) are particularly well-suited for the classification due to their ability to capture spatial-spectral patterns. AVariational Autoencoder (VAE) CNN model will be developed to enable unsupervised learning to efficiently encode hyperspectral data and extract spectral features and fractional abundances. The project will train intern students to collect hyperspectral radiometer measurements from field and lab experiments and characterize water sample spectra (Fig. 1 a-c). Techniques such as chlorophyll-a and harmful algae bloom indices (Fig. 1d-g) will be used to characterize water constituent variations. The Remote Sensing Laboratory activities will foster a broader scope of collaboration and engagement between the UMD/CISESS team and the NOAA sensor calibration team.The insights gained from this project will be instrumental in preparing for future hyperspectral imaging sensors.
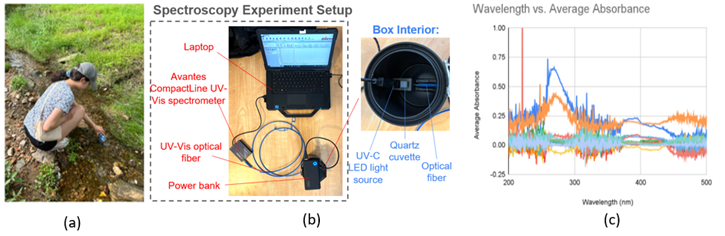
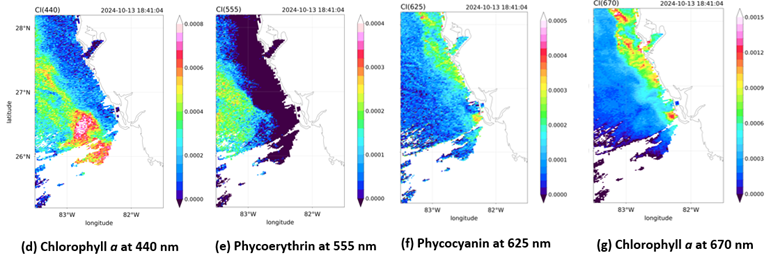
Figure 2: (a) Field experiment of water sample collection. (b) Lab experiment setup for water spectroscopy measurements. (c) Measured absorbance spectrum for water samples from different locations.(e-h) Preliminary index maps over Florida coastal region after Hurricane Milton for Chlorophyll a (440 and 670 nm) and pigments (Phycoerythrin and Phycocyanin) from the tri-band absorption spectral analysis. It confirmed the algae bloom on Oct. 13,2024.
« Back