Proactive Quality Control
Daisuke Hotta 1,2, Eugenia Kalnay 1, Yoichiro Ota2
1 University of Maryland, 2 Japan Meteorological Agency
Numerical Weather Prediction (NWP) has gone through remarkable advances over the recent few decades. Exploitation of satellite-based remote-sensed data, along with the introduction of sophisticated data assimilation methods such as variational techniques and ensemble Kalman Filtering (EnKF), has played an important role. Although the NWP boasts impressively high forecast skills on average, it occasionally suffers from abrupt drop of performance. In order for a better operational reliability, NWP centers have to minimize the occurrences of such forecast skill “dropouts”. Recent studies have shown that those “dropouts” occur not because of the model’s deficiencies but because of the assimilation of flawed observations that the operational quality control (QC) system failed to filter out. The major challenge in preventing such “dropouts” resides thus in detecting those “flawed” observations.
A diagnostic technique called Forecast Sensitivity to Observation (FSO) enables us to quantify how much each observations improved/degraded the forecast. Our group at University of Maryland has succeeded in devising an ensemble-based formulation of FSO, which we call EFSO, and have successfully implemented it to a quasi-operational global ensemble data assimilation system coupled with NCEP’s GFS model (Kalnay et.al 2012; Ota et.al 2013). While most previous FSO studies, which use an adjoint-based formulation, have been predominantly concerned with statistical properties of observational impact, Ota et.al (2013) successfully demonstrated that it is possible, for individual cases, to identify “flawed” observations that are responsible for forecast skill dropouts by applying EFSO with 24-hour forecast lead-time to a relatively small horizontal region. Figure 1 shows the case of a successful identification of flawed observations (MODIS winds near the North Pole): the 24-hour forecast exhibits a sharp dipolar error near the Pole (leftmost panel). EFSO diagnostics applied to the area indicated by the magenta cone identified the MODIS winds in that area as the suspicious observations. The EFSO estimated change of the forecast by not assimilating these observations (center panel) nearly perfectly cancels the dipolar error that is present in the forecast error. In fact, the actual change of forecast by denial of the identified MODIS winds (rightmost panel) is almost identical to the estimation by EFSO and it reduces regional forecast error by as much as 30%.
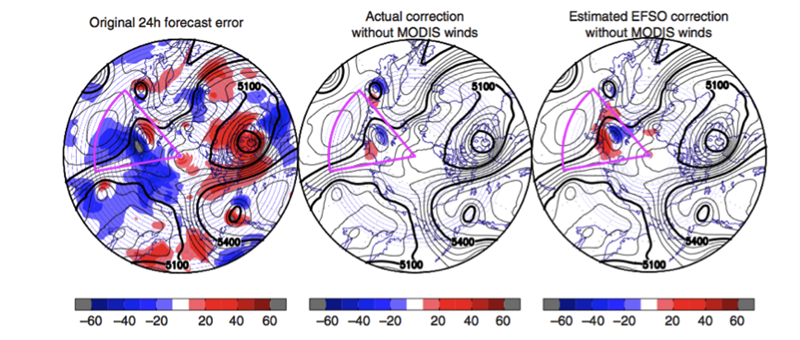
Figure 1: (from Ota et.al 2013, Fig. 9) Twenty-four hour forecast error of 500 hPa geopotential height (unit: m, 18 UTC 6 February 2012 initial) from original analysis (left) and forecast change due to the removal of the MODIS polar wind observations in the data-denial experiment (middle: actual change and right: projection on the ensemble perturbations). Black contours show the analysis. Magenta cones show the target area of the observation impact estimate.
The “Proactive QC”, our proposed new QC scheme, exploits this EFSO’s capacity to detect defective observations that actually degraded forecast skill: we first perform data assimilation using all the available observations that passed the standard operational QC. Then, 6 hours later, when the analysis at the next cycle becomes available, we compute regional 6-hour forecast errors and apply an algorithm to detect “regional skill dropouts”. We next carry out EFSO diagnostics on those detected regions to identify observations that are likely to have significantly degraded 6-hour forecasts. If such “flawed” observations are identified, we repeat the data assimilation without using the identified observations.
Concerns that need to be addressed before adapting Proactive QC into the operational system include whether it also works for ensemble/variational hybrid data assimilation system and whether the lead-time of 6 hours is long enough to capture meaningful signals of the forecast errors. In order to address these issues, we have implemented the EFSO diagnostics tool to a lower-resolution version of the operational ensemble/3D-Var hybrid GSI and tested EFSO diagnostics with three different forecast lead-times (6, 12 and 24 hours). We have found that, contrary to the concerns stated above, EFSO gives consistent results independent of the data assimilation system used (pure ensemble or hybrid) and of the choice of forecast lead-time, not only in terms of statistical properties but also for individual cases. Figure 2 shows the impacts of observations to the forecast classified based on the types of observations for the case shown in Figure 1. Positive values correspond to increase of forecast errors (i.e., negative impacts). This figure shows that strong negative impact from MODIS wind in this region can be clearly identified even with a lead-time as short as 6 hours. This not only shows that our new QC algorithm is feasible but also corroborates the robustness of our approach.
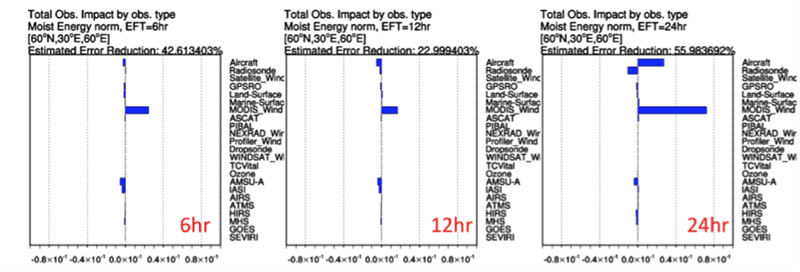
Figure 2: Estimated forecast error reduction contributed from each observation types for lead-times of (left) 6 hours, (middle) 12 hours and (right) 24 hours for 18 UTC 6 February 2012 initial. The forecast errors are measured with the moist total energy norm (unit: J kg-1) for the area of [60°N-90°N, 30°E-60°E].
Our proactive QC is a major innovation because it will allow us, for the first time ever, to carry out fully flow-dependent QC based on whether the observation actually degraded the forecast. Another significant outcome of our Proactive QC is that it would enable us to build a detailed database of failed observations by collecting all their occurrences along with relevant metadata; such database can then be provided to algorithm developers to help them to correct the problem that produced the bad observations and avoid them in the future. Finally, the Proactive QC will allow a more efficient and precise determination of the optimal way to assimilate new observing systems. The current approach, based on comparisons of forecasts started from “experiment” and “control” analyses (made with and without using the new observations) has difficulties obtaining statistically significant results in the presence of the rest of the available observing systems. The Proactive QC should address this problem by finding the short-term impact of each observation and allowing the comparison of the impact of different observation algorithms.
References
Kalnay, E., Y. Ota, T. Miyoshi and J. Liu, 2012: A simpler formulation of forecast sensitivity to observations: application to ensemble Kalman filters, Tellus A, 64, 18462, http://dx.doi.org/10.3402/tellusa.v64i0.18462
Ota, Y., J. C. Derber, E. Kalnay and T. Miyoshi, 2013: Ensemble-based observation impact estimates using the NCEP GFS, Tellus A, 65, 20038, http://dx.doi.org/10.3402/tellusa.v65i0.20038
« Back